During this cleanse process, we combined the preliminary set of images collected from recipe websites and the new ones collected through Google image search. Then within the second part, we augmented every recipe in this preliminary collection with food pictures downloaded from the web utilizing a well-liked picture search engine, which amounted to over 13M meals images after cleansing and eradicating actual-and-close to duplicates. Data Download....
Jumat, 03 Juni 2022
Kamis, 02 Juni 2022
What Does Recipe Mean?
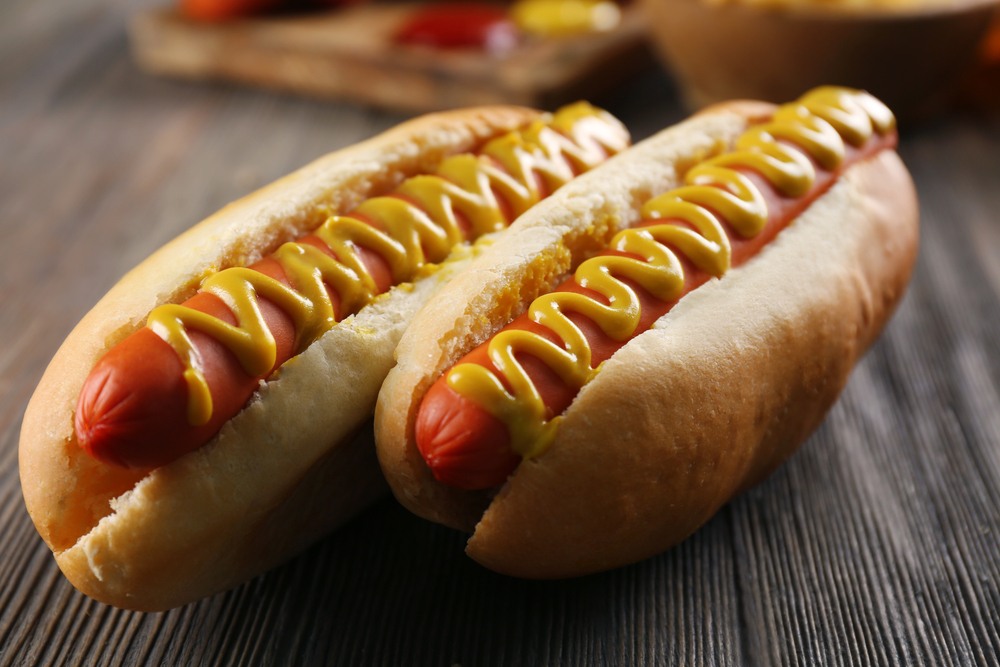
If recipe instructs you to beat egg whites until stiff peaks form, you possibly can check by lifting beaters straight up from egg whites. An homage to what it means to be Korean American with delectable recipes that discover how new culinary traditions may be forged to honor both your previous and your present. She pored over Korean prints from the Joseon dynasty, trying to seize the era’s kitchens and serving traditions. And take into consideration...
Rabu, 01 Juni 2022
Poll: How A lot Do You Earn From Recipe?
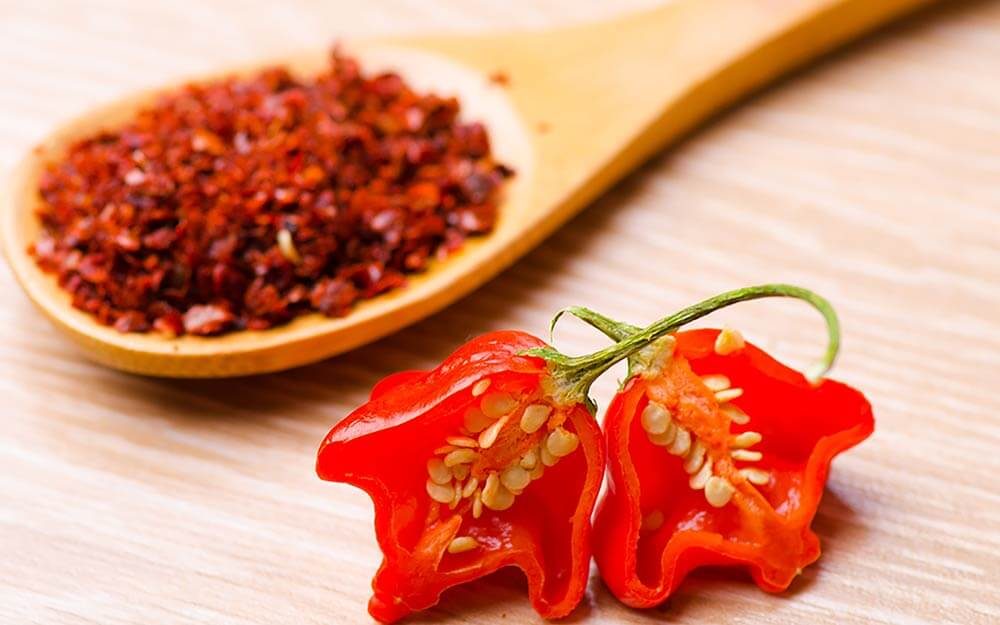
First, in Sect. 2 we describe in additional element the elements our recipe requires. It is a basic recipe to assemble quasi triangular Hopf algebras from a given Hopf algebra. For convenience we solely work with the case of finite groups though our strategies may be extended to the case of steady compact groups and normally to involutory Hopf algebras. Thus the quantum double Hamiltonian realizes a illustration of the quantum double of these involutory...
Selasa, 31 Mei 2022
Korean Food Tip: Be Consistent
Halo Top's new recipe is rolling into shops now. Most of us don't have to travel far to find a roast rooster; they are often found in all places from carry-out shops and casual dining institutions to small grocery shops and big food warehouses. The profusion of on-line recipe collections with person-submitted images presents the possibility of training machines to mechanically understand food preparation by jointly analyzing ingredient lists, cooking...
Minggu, 29 Mei 2022
Take The Stress Out Of Recipe
On the recipe facet, as proven in Figure 5, the attention model learns to deal with components which might be interpreted based mostly on visible connections with the meals photos. This radius is determined by numerical integration of the most effective match mannequin Sérsic operate from the 2-element decomposition. The most effective similarity measure is determined by the necessities of a specific utility. Ideally, the best procedure is to immediately...
Sabtu, 28 Mei 2022
Six Ways You'll Be Able To Eliminate Recipe Out Of Your Enterprise
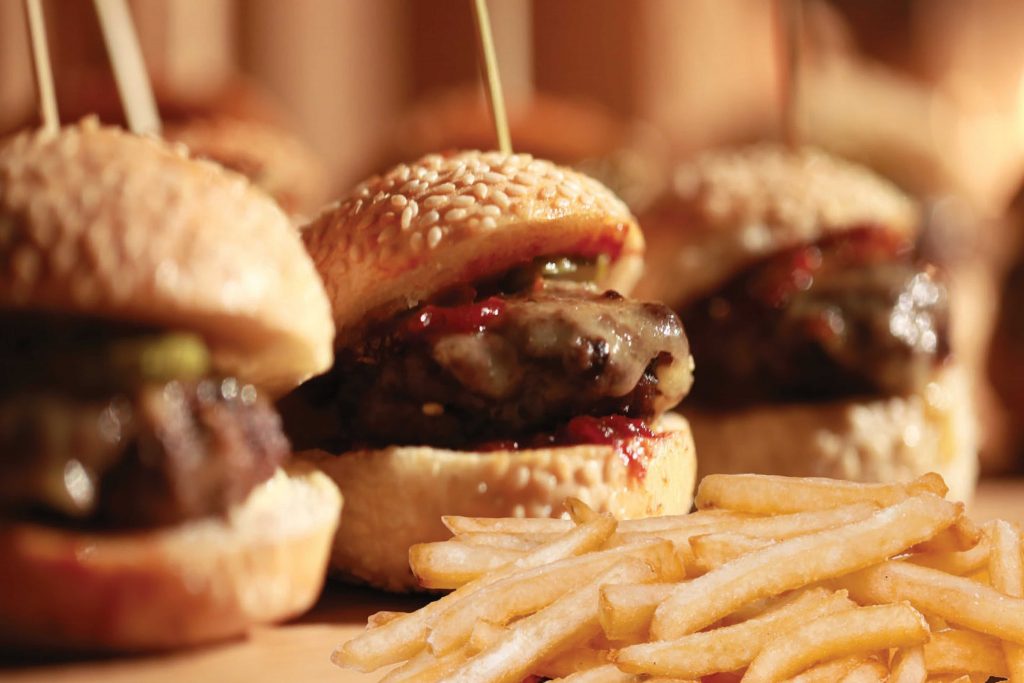
POSTSUBSCRIPT, however there is yet a drastic simplification to be achieved earlier than we reach our final recipe as we are going to see in the next part. Find out easy methods to throw the ultimate holiday bash from start to finish using the information and solutions in this article. It turns out that the restriction to the Einstein condition on the boundary metric does not spoil the independence of the curvature invariants of sort-A and type-B...
Jumat, 27 Mei 2022
Remarkable Website - Recipe Will Enable You Get There
Most of the present fashions that use recipe data purpose to advocate recipes to customers based mostly on earlier scores of other users and recipes. This suggestion system will treat recipes as users, while the ingredients will play the role of the items these recipes have ‘rated’. Furthermore, we are going to explore the advantages of applying dimensionality reduction strategies to deal with sparse binary knowledge. We've opted for experimenting...